Web3’s Personalization Playbook: Lessons to Learn from Netflix
By Alev • August 30, 2024
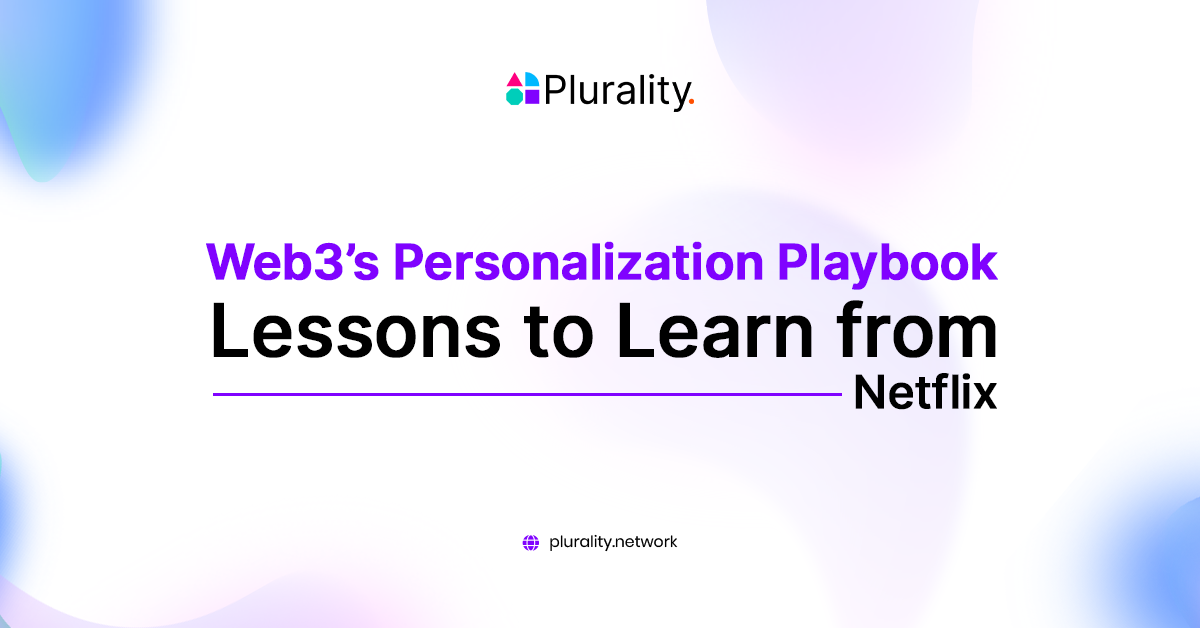
Personalization has become the talk of the town in recent times, as we have many streaming platforms and other evolving web ecosystems. So, what exactly is personalization, and how has it become a big deal?
Personalization is tailoring content, services, and experiences to meet individual users’ preferences and behaviors.
This concept has dramatically influenced how content is delivered across devices and platforms. One of the most successful personalization implementations is Netflix’s recommendation system, which only keeps improving.
By capitalizing on rich user data sources, Netflix has redefined content consumption into bespoke experiences that assure high levels of engagement and stickiness.
However, as new decentralized streaming platforms (like Livepeer and Tape) are now in play, the way such a level of personalization will be achieved is filled with new challenges and opportunities.
This article will explore the mechanics of Netflix’s personalization model, how data is essential to that process, and the complications of executing such strategies within a framework like Web3.
The Inner Mechanics of the Netflix’s Personalization Engine
The Netflix recommendation model works in harmony between algorithm and data science to make recommendations using machine learning and AI for a user’s taste. Key components of the recommendation system at Netflix include:
Collaborative Filtering: This technique identifies user behavior patterns, such as viewing history and ratings, that are interested in suggesting content similar users enjoy. Using the clustering process, the system groups users with shared preferences and thus enables Netflix to recommend shows and movies popular among peers with analogous tastes.
Content-Based Filtering: This technique assesses content attributes, including genre, cast, director, and metadata such as description and tags. For any user, Netflix looks at the properties of content users have watched and recommends other titles with similar properties.
Deep Learning Algorithms: Netflix leverages neural networks to make much finer adaptations to the recommendation system. Primary datasets stream through these algorithms to give more profound user preference and content similarity that can be very difficult to notice with simpler models.
Contextual and Behavioral Analysis: Besides explicit user inputs such as ratings, Netflix considers a host of implicit signals, including viewing time, device type, and viewing location. These data sets inform and refine recommendations to make them relevant to a user’s context.
The combination of these algorithms results in a dynamic recommendation engine that evolves based on changes in users’ preferences over time. This would be an essential step toward continuous refinement, the key to user engagement and satisfaction.
Economic Value of Personalization
Personalization is not a feature at Netflix but rather a strategic asset at its core, powering its business model. Some of the significant economic values of Netflix personalization include:
Increased User Retention: It is challenging for users to quit when they find something interesting regularly. According to different reports, about 80% of Netflix viewed content is discovered through its recommendation engine; hence, the power of personalized content delivery underlines the retention of subscribers.
Improved User Acquisition: Netflix’s ability to show value quickly through a personalized experience is crucial to its success, especially during the critical onboarding phase. When a new user signs up, Netflix leverages its robust recommendation engine to offer content suggestions that align closely with the user’s potential interests.
This tailored experience is crucial within the first few seconds of the user journey—often called the “7-second rule“- the brief window in which a user decides whether to engage further with a platform or leave. By immediately presenting relevant content, Netflix increases the likelihood of converting free trials into paying subscriptions, turning curiosity into commitment.
Better Content Investment: User data would enable Netflix to decide what new content to produce or acquire. This would minimize the risks associated with content investment while increasing the ROI.
According to industry reports, the recommendation system saves Netflix around $1 billion annually through reduced churn and higher user engagement. This shows how crucial personalized experiences have become for digital platforms aiming for sustainable growth.
Challenges with Personalization in Web3
Whereas personalization has proved a powerful vehicle for engaging users in Web2, deploying it in web3 brings about some very particular difficulties arising from the very nature of the differences in data management and processing:
Decentralization of Data: While on Web2, the data rested very centrally with platforms like Netflix, which could warehouse and analyze it en masse, fundamentally, Web3 rests on decentralization where:
(a) most of the data available concerns financial transactions and not behavioral or interest data, and
(b) data is scattered over networks. This is what makes aggregation and analysis necessary for personalization so complicated.
Web3 privacy and user sovereignty highlight sharp contrasts with the very foundation of data collected in typical Web2 personalization. In a decentralized environment, users must agree to use shared data, and platforms must find a way to provide personalized experiences without compromising user privacy or control.
Technical Limitations: Currently, all existing Web3 technologies, including blockchain and decentralized storage solutions, need to be optimized to deal with large-scale data analytics in real-time, representing one of the critical requirements behind such dynamic personalization featured on platforms like Netflix.
Such limitations require the development of new technologies and frameworks to support data processing while keeping privacy efficient.
Personalization in Web3 must be redesigned, finding creative approaches built with decentralized technologies where users still benefit. Possible ways to address this might be:
Federated Learning: A decentralized machine learning technology allows algorithms to be trained across multiple devices or nodes without aggregating data into a central location.
Every user’s local device will process some of the data locally, while only the model updates, not the raw data, are shared across the network. This preserves user data privacy and supports personalization in the platform.
Zero-knowledge proofs enable one party to prove to another that some statement is true without leaking information other than the truth. ZKPs might allow Web3 platforms to validate user preferences or behaviors required for personalization without touching the raw underlying data.
Decentralized Identity Systems: DID systems give users ownership of their digital identities and, by extension, the data from those identities across various platforms. Users can selectively share data points necessary for personalization while maintaining complete control over their information. This fits nicely into the Web3 ethos around data sovereignty.
Privacy-Preserving Computation: Homomorphic encryption and secure multi-party computation allow for certain types of data processing in encrypted form. It may be possible to run complex personalization algorithms on decentralized networks without leaking sensitive user data.
Plurality Network’s Role in Web3 Personalization
At Plurality Network, we are building the infrastructure necessary to make these new paradigms of personalization real. Our platform is designed to bridge the gap between the demands of the personal experience and the need for privacy and user control in Web3.
Plurality Network empowers a robust ecosystem whereby personalized experiences can thrive without sacrificing user sovereignty. This is possible thanks to combining technologies like federated learning, decentralized identities, and privacy-preserving computation.
Conclusion
Personalization was and will be a significant driver of digital engagement, as shown by Netflix’s success in utilizing customer data to enhance the nature of the viewing experience. However, as the digital world moves forward and decentralizes with Web3, strategies and technologies need to change to achieve such a level of personalization.
There are several ingenious solutions to decentralization challenges, privacy, and technical limitations that can keep up with the high level of user engagement that personalization offers.
At Plurality Network, we are committed to pioneering these solutions so that Web3 can deliver the personalized experiences users require without sacrificing the principles of privacy and decentralization.
By reimagining personalization within the context of Web3, we’re building a better user experience and the building blocks for a more equitable and user-centric Internet.